Insights
Face recognition based on the analysis of 3D scans has been an active research subject over the last few years. However, the impact of the resolution of 3D scans on the recognition process has not been addressed explicitly, yet being an element of primal importance for the introduction of a new generation of consumer depth cameras. These devices perform depth/color acquisition over time at standard frame-rate, but with a low resolution compared to the 3D scanners typically used for acquiring 3D faces in recognition applications.
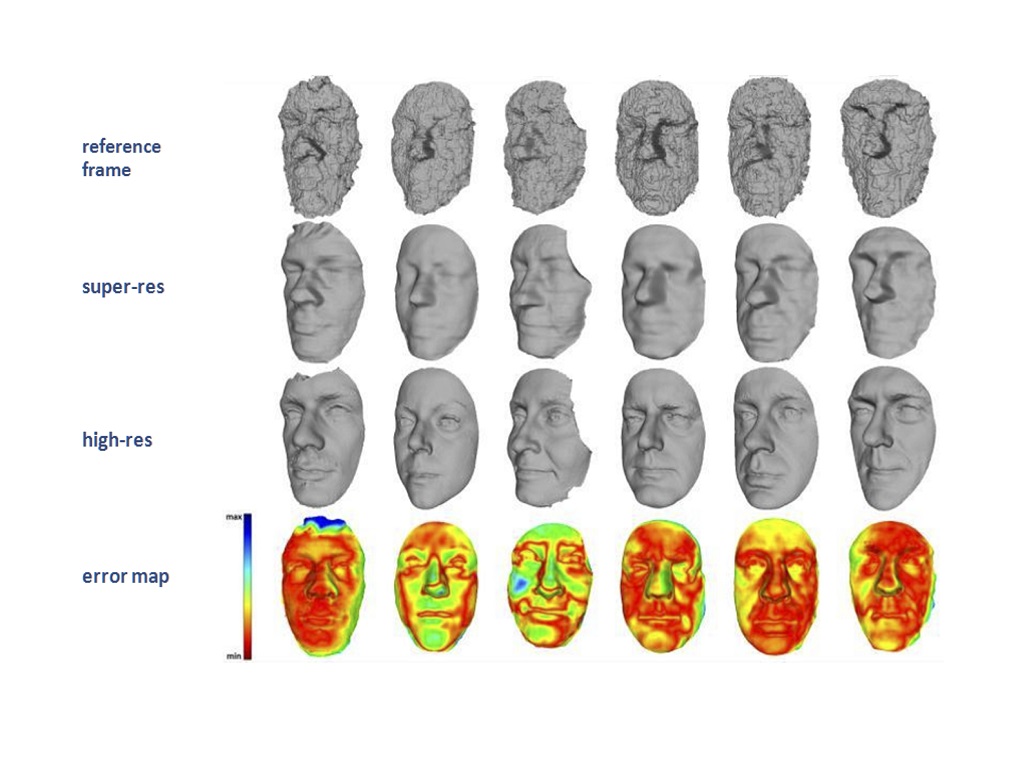
Motivated by these considerations, in this project we define a super-resolution approach for 3D faces by which a sequence of low-resolution 3D scans is processed to extract a higher-resolution 3D face model. The proposed solution relies on the Scaled ICP procedure to align the low-resolution scans with each other, and estimates the value of the high-resolution 3D model through a 2D Box-spline functions approximation.
The proposed approach relies on scattered data approximation techniques and operates in three main processing steps: i) First, for each depth frame of the sequence, the region containing the face is automatically detected and cropped; ii) Then, the cropped face of the first frame of the sequence is used as reference and all the faces cropped from the other frames are aligned to the reference; iii) Finally, data obtained by aggregating these multiple aligned observations are resampled at a higher resolution and approximated using 2D-Box splines.
To validate the proposed approach and estimate the accuracy of the reconstructed super-resolved models, the The Florence Superface v2.0 dataset has been constructed. For each individual, the dataset includes one sequence of depth frames acquired through a Kinect scanner as well as one high-resolution face scan acquired through a 3dMD scanner. In this way, the accuracy of the reconstructed super-resolved model can be quantitatively measured by comparing the reconstructed model to the corresponding high-resolution scan.