Insights
Location-Based Social Networks (LBSNs), with their huge amount of geo-located user generated content, are providing a lot of semantics on human mobility and behaviour as well as on users’ interests and activities in cities .
LiveCities is a web application with the purpose to reveal the hidden character of cities through check-ins and venues’ clustering and information visualisations paradigms. The main novelty in the system is constituted by the way feature selection is performed. Venues are analysed on the basis not only of check-in categorisation but also considering the semantics of Facebook profiles of people who checked-in.
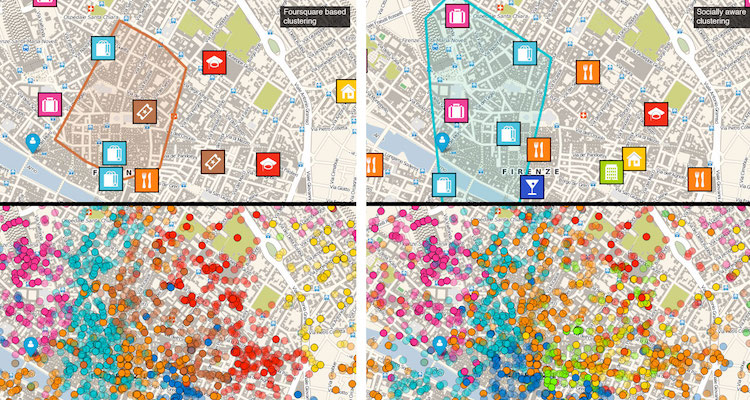
Furthermore LiveCities provides personal recommendation of areas and venues matching user interests and allows the free exploration of urban social dynamics in terms of people lifestyle, business, demographics, transport etc. with the objective to uncover the real ‘pulse’ of the city.
The application has been developed in Java as a web servlet and deployed in the Apache Tomcat container. The backend is composed by three modules: a servlet for collecting the initial dataset from Facebook, the web application and a module for the execution of clustering and recommendation algorithms.
We conducted a preliminary evaluation of the results obtained by the clustering algorithm in order to asses if the proposed feature selection method performs better than other common approaches.
A group of citizens of the city of Florence (IT) were asked how they would ‘label’ city areas according to their perception and then to compare the results with the clustering output.
Results show that the proposed feature selection for clustering city venues (socially aware), based on implicit semantics extracted by user profiles, has a lower error rate than the other based on geographical and Foursquare based features.