Insights
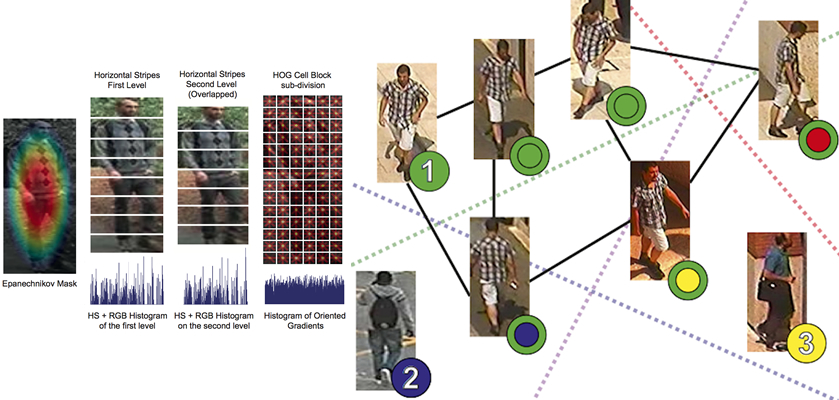
At MICC we have several research lines addressing the Person Re-Identification problem. Our contributions to the state-of-the-art in person re-identification are:
- Re-identification by discriminative, sparse basis expansion: We introduced an iterative extension to sparse discriminative classifiers capable of ranking many candidate targets. The approach makes use of soft- and hard- re-weighting to redistribute energy among the most relevant contributing elements and to ensure that the best candidates are ranked at each iteration. Our approach also leverages a novel visual descriptor (WHOS) based on coarse, striped pooling of local features which is both discriminative and efficient. It exploits a simple yet effective center support kernel to approximately segment foreground from background. The proposed descriptor is robust to pose and illumination variations, and jointly with our sparse discriminative framework yields state-of-the-art results.
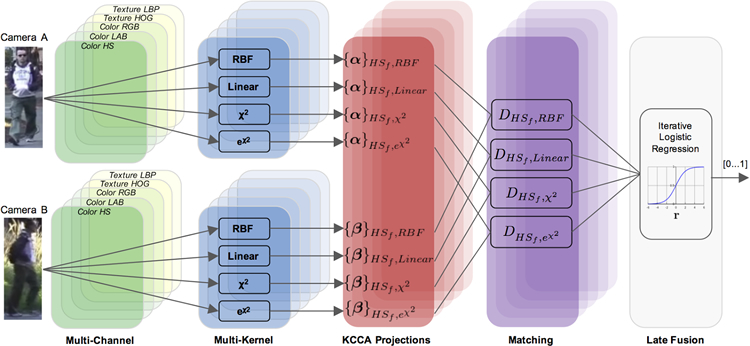
- Semi-supervised large-scale person re-identification: We have also proposed a semi-supervised approach that combines discriminative models of person identity with a Conditional Random Field (CRF) to exploit the local manifold approximation induced by the nearest neighbor graph in feature space. The linear discriminative models learned on few gallery images provides coarse separation of probe images into identities, while a graph topology defined by distances between all person images in feature space leverages local support for label propagation in the CRF. Discriminative model and the CRF are complementary and the combination of both leads to significant improvement over the state-of-the-art. The performance of our approach improves with increasing test data and also with increasing amounts of additional unlabeled data.
- Person re-identification across camera: In this work we showed how several color and texture features are extracted from a coarse segmentation of the person image can account for viewpoint and illumination changes. For each feature, we learn several projection spaces where features computed on images of the same person observed in two different cameras correlate. These projection spaces are learned using Kernel Canonical Correlation Analysis (KCCA). Matching between images from two cameras is performed by applying an iterative logistic regression procedure that enables selecting and weighting the contributions of the distances computed in each projection space.