This talk presents our research on multiview, articulated human motion tracking from its origins within pose estimation for immersive communications, through its evolution to full-body, model-free tracking using evolutionary search, to our current system. In the latter, we capture synchronized sequences of single-person activities (e.g., walking, kicking, punching) in our 10-camera, green-background studio.
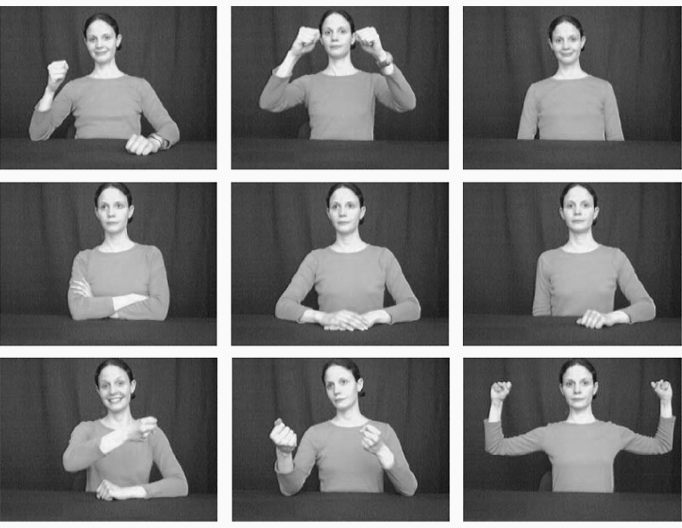
Articulated human hotion tracking with latent spaces and evolutionary search
Instantaneous frames are segmented and silhouettes represented with shape contexts. Silhouette representations, computed for the whole sequence, are converted into a low-dimensional latent space by charting, a dimensionality reduction technique not used before for human motion tracking.
A supervised training phase learns a manifold in latent space for each action (the action model). Generative tracking takes place in the latent space. Pose hypotheses are evaluated without expensive backprojecting to 3-D space, avoiding the costly generation of synthetic silhouettes; instead, a mapping between latent and silhouette space is learnt off-line for each action modelled. Results indicate state-of-the-art performance for the actions tested, at very modest computational costs compared with similar systems.
Current investigations include on-line action recognition and applications to clinical rehabilitation. Key contributors to the research described were Spela Ivekovic, Vijay John, and Craig Robertson.