Semantic image segmentation is the process of assigning semantically relevant labels to all pixels in an image. Hierarchical Conditional Random Fields (HCRFs) are a popular and successful approach this problem. One reason for their popularity is their ability to incorporate contextual information at different scales. However, existing HCRF models do not allow multiple labels to be assigned to individual nodes. At higher scales in the image, this results in an oversimplified model, since multiple classes can be reasonable expected to appear within a single region. This simplified model especially limits the impact that observations at larger scales may have on the CRF model. Furthermore, neglecting the information at larger scales is undesirable since class-label estimates based on these scales are more reliable than at smaller, noisier scales.
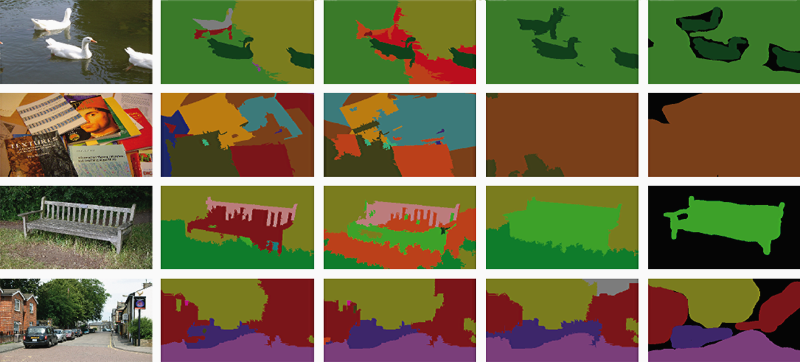
The harmony potential: fusing local and global information for semantic image segmentation
In this talk I will discuss a new potential function, the harmony potential, for defining HCRF models of semantic image segmentation. The harmony potential can encode any possible combination of class labels at the global level, enabling it to make better informed, fine discriminations at the low levels. This representational capacity of the harmony potential is also its primary weakness as the optimization over all possible labels quickly becomes intractable for more than a few classes. To address this, we show how the harmony potential model admits an effective sampling strategy that renders tractable the underlying optimization problem. Results show that our approach obtains state-of-the-art results on two challenging datasets: Pascal VOC 2009 and MSRC-21. The approach described in this talk additionally won six gold medals in the Pascal VOC 2009 Segmentation Challenge.